What are the challenges and solutions associated with AI bias? The answer is that AI bias stems from diverse sources ranging from data to human involvement, affecting outcomes and reliability. However, strategies like diverse datasets and algorithm transparency can mitigate these biases.
June 30, 2024 (4mo ago) — last updated September 13, 2024 (1mo ago)
Understanding AI Bias: Challenges and Solutions
AI bias can have significant consequences. This article explores the roots of AI bias and provides solutions for creating fairer AI systems.
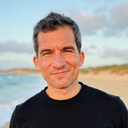
What is AI Bias and Where Does It Come From?
Artificial Intelligence (AI) has revolutionized countless industries, yet it’s not free from drawbacks. One of the most concerning issues is AI bias. But what causes AI bias, and why should we care?
AI bias occurs when an AI system exhibits partiality due to prejudices ingrained during its lifecycle. These biases can emerge from various sources and impact the fairness and accuracy of the system's outputs.
The Roots of AI Bias
AI bias is multifaceted, originating from:
- Training Data: Biased datasets reflect existing societal prejudices, leading AI to "learn" and perpetuate these biases.
- Algorithm Design: Developers' own conscious or unconscious biases may influence the algorithms they create.
- Inequitable Representation: Failure to include a diverse range of inputs can skew AI's predictions and decisions.
Why AI Bias Matters
The implications of AI bias are vast and significant:
- Social Inequality: Biased AI systems can reinforce and even exacerbate social inequalities.
- Incorrect Decisions: Inaccurate predictions can have serious repercussions, especially in areas like healthcare and criminal justice.
- Loss of Trust: When AI systems are perceived as unfair, it erodes public trust in technology and its potential benefits.
Tackling AI Bias: Practical Solutions
So, how can we create more equitable AI systems? Here are several strategies:
Diverse and Representative Datasets
Ensuring your training data encompasses a broad spectrum of demographics and scenarios is crucial:
- Audit and Clean Existing Data: Identify and remove biased data points.
- Inclusive Data Collection: Actively seek out diverse data sources.
Transparent Algorithm Development
Promoting openness in how AI algorithms are designed and refined helps everyone understand and address biases:
- Explainability: Develop algorithms that can explain their decision-making processes.
- Independent Audits: Regularly assess algorithms through independent reviews to identify biases.
Continual Monitoring and Feedback Loops
Bias mitigation isn’t a one-time task but a continuous effort:
- Post-Deployment Monitoring: Continuously analyze AI outputs for signs of bias.
- User Feedback: Encourage and incorporate feedback from diverse user groups to refine AI performance.
Relevance to OneTask
As an AI-powered personal admin assistant, OneTask prioritizes fairness and accuracy. By leveraging best practices such as diverse data and transparent algorithms, OneTask ensures reliable and unbiased task management and scheduling assistance for all users.
In addition, OneTask's ongoing commitment to ethical AI development means users can trust that their AI assistant will operate equitably, adapting to a wide range of personal preferences and professional needs.
Further Reading
To expand your understanding of AI and its transformative potential, check out these related posts:
- AI Deep Learning: Explore how deep learning techniques shape AI's ability to handle complex tasks.
- AI Emergent Behavior: Learn about emergent behaviors in AI and their broader implications.
- Empower Your Product Marketing Strategy: Top Books You Need: Discover essential readings that can enhance your product marketing strategy, which is crucial for understanding the market dynamics that influence AI development.
AI bias is a critical challenge, but by implementing conscious strategies, we can work towards creating more equitable and trustworthy AI systems. At OneTask, we’re committed to leading the way in this ethical endeavor.
Join OneTask Today!
Unlock your productivity potential with OneTask. Sign up now and start managing your tasks efficiently.
ONETASK